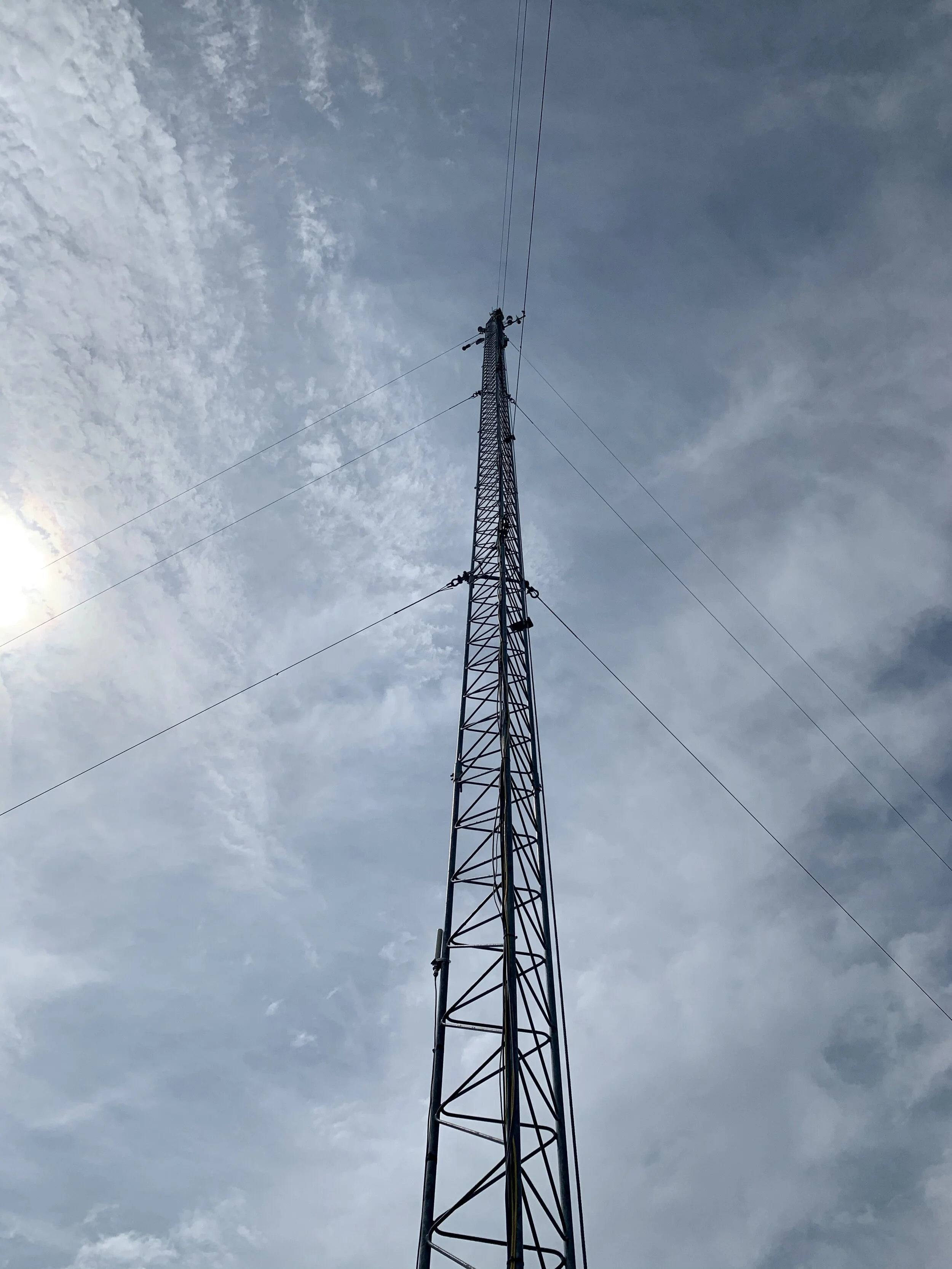
Improving Lifecycle Analyses of Crop-Livestock Systems Using Remote Sensing And Eddy Covariance Data
This project uses eddy covariance techniques and remote sensing data, from satellites (Landsat, MODIS, Ecostress) and drones, to estimate farm vegetation productivity, to improve greenhouse gas budgets for agricultural systems. When we look at lifecycle analyses, especially in livestock systems, were are often only confronted with emission estimates, while ignoring how the vegetation can help mitigate some of the emissions produced on farm. Sustainably managed crops, especially perennial vegetation, takes up atmospheric CO2 and stores it in above- and below ground biomass. Optimal management, such as no till and conservative fertilizer or manure applications, can then help sequester that carbon in the soil. This ecosystem service and others can then be used as extra profits for farmers, using ecosystem credit markets, that will pay the producer for these services.
We collect several ground measurements to help validate our eddy covariance and remote sensing data, which include above- and below ground biomass sampling, soil respiration, vegetation indices, and soil texture, infiltration and nutrient sampling, which includes microbes. These data help verify the remote sensing data, but also help us understand how cropping management affects nutrient cycling and soil quality in these systems. Healthy soils will store more carbon, are less prone to pathogens, require less inputs like fertilizer and reduce nutrient runoff, an important consideration for Wisconsin, where extreme precipitation events are common.
I am currently working on quantifying how the combination of eddy covariance data from agricultural systems and remote sensing data can help decrease the uncertainty from estimating such greenhouse gas budgets. We are also quantifying how cropping management affects soil respiration and how this relates to microbial diversity and soil nutrient cycling.
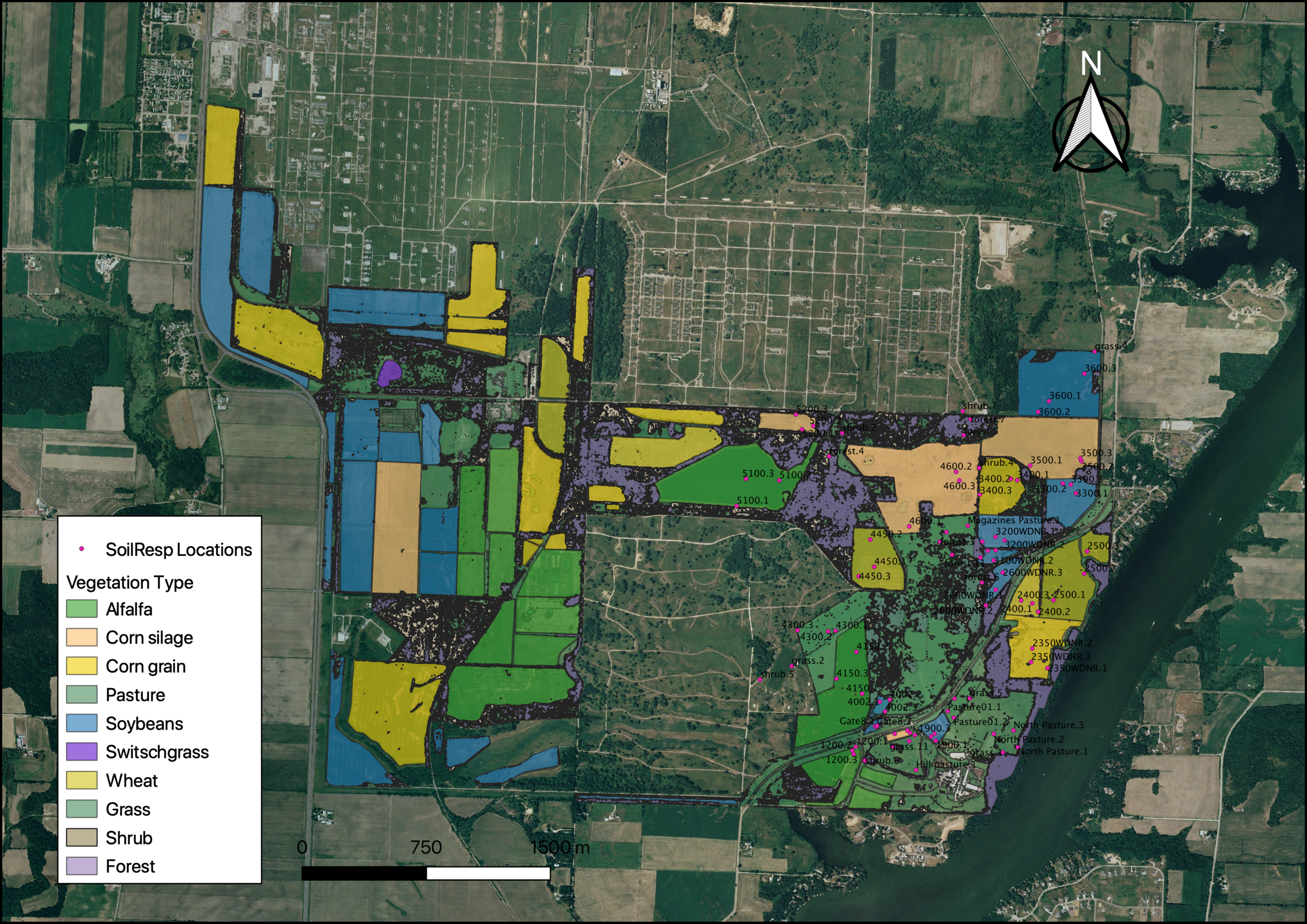
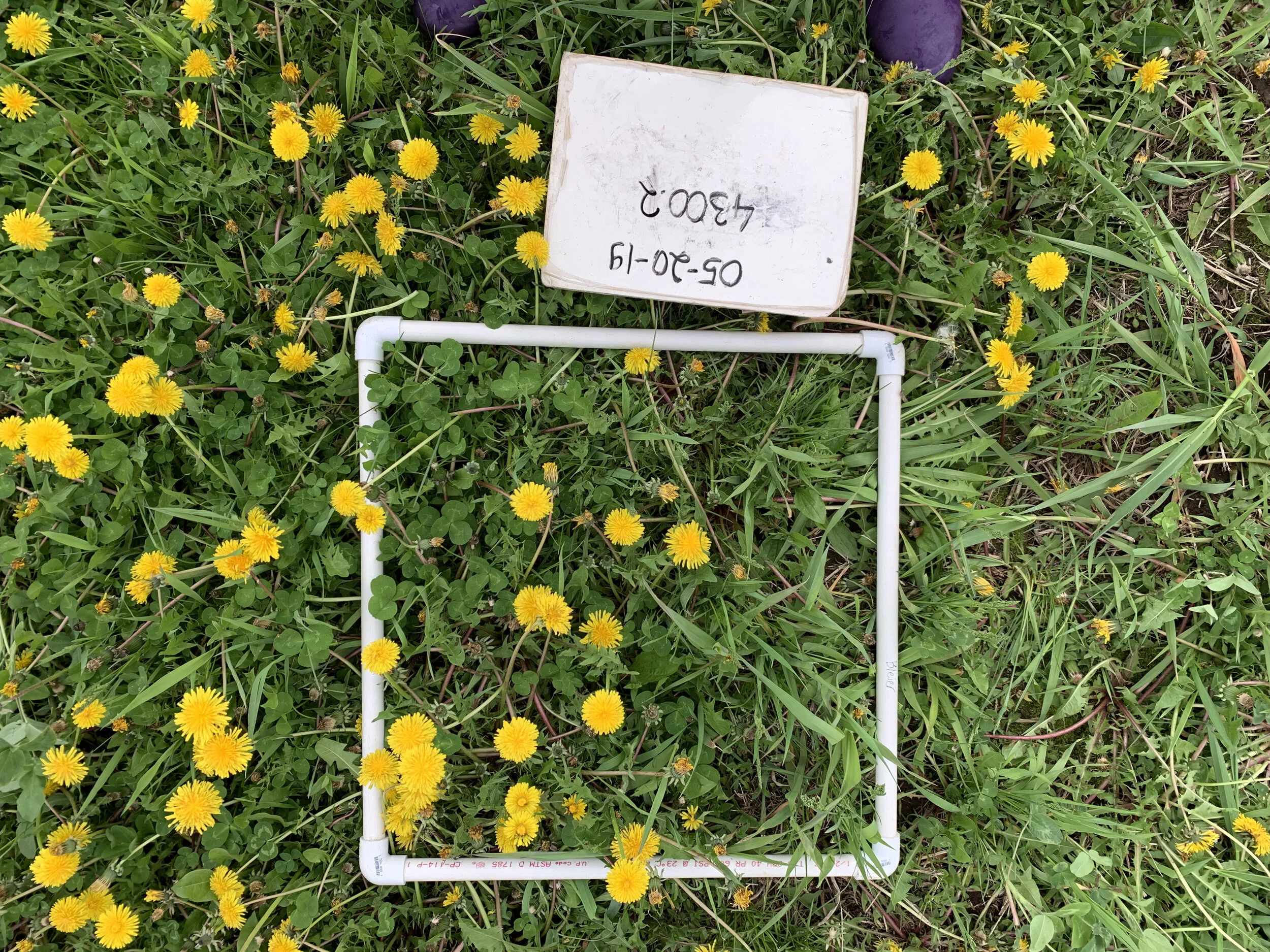
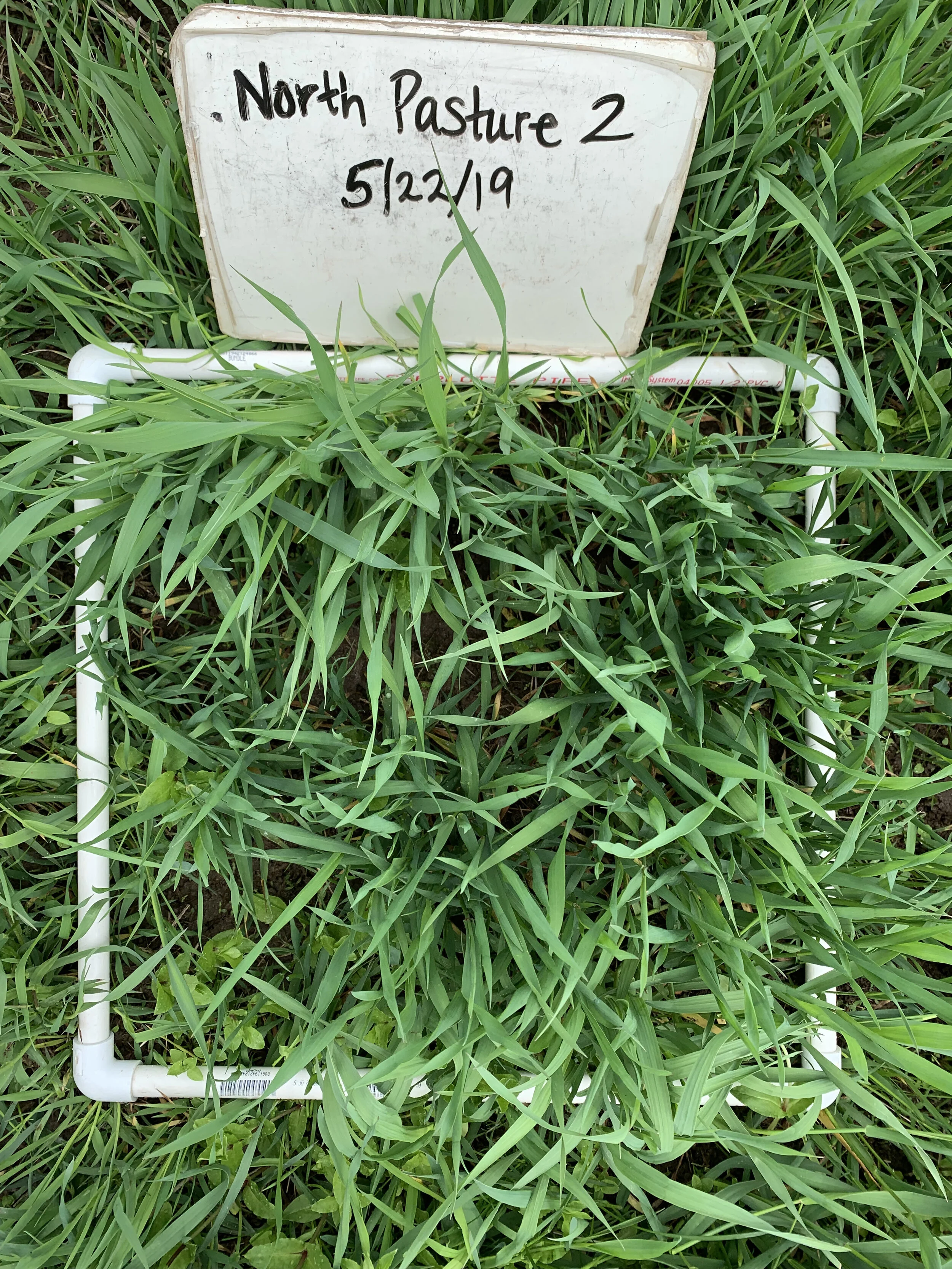
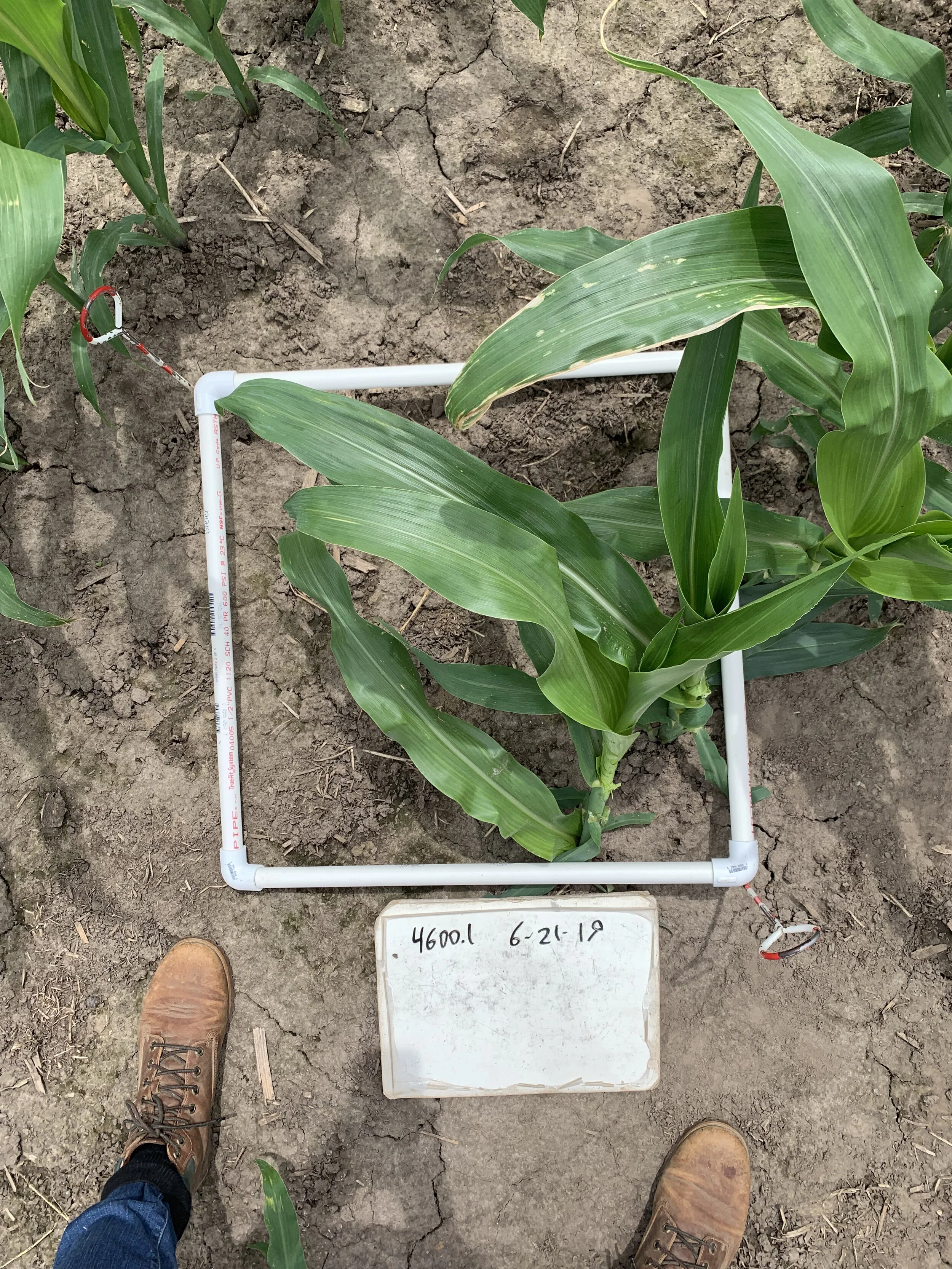
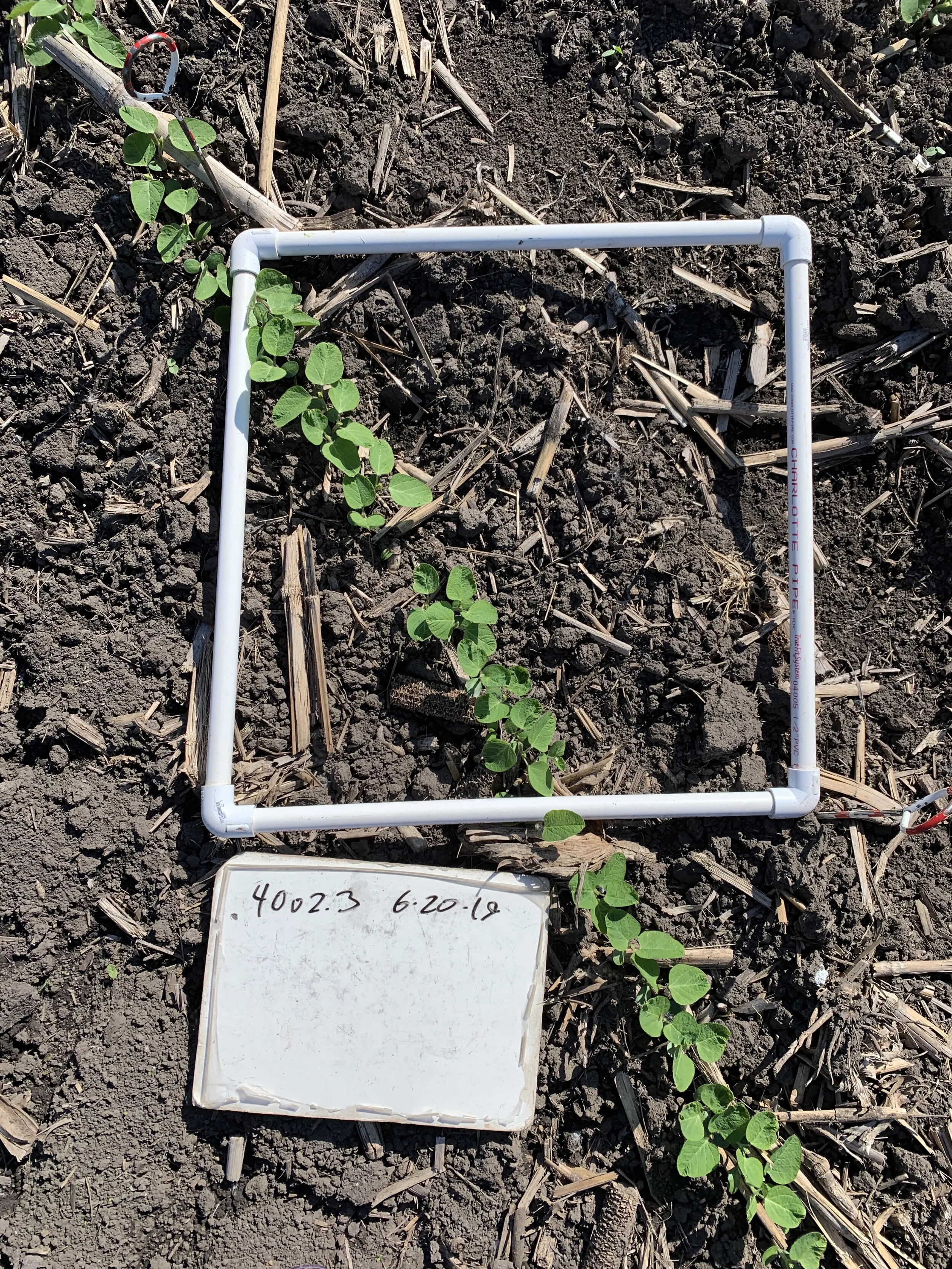
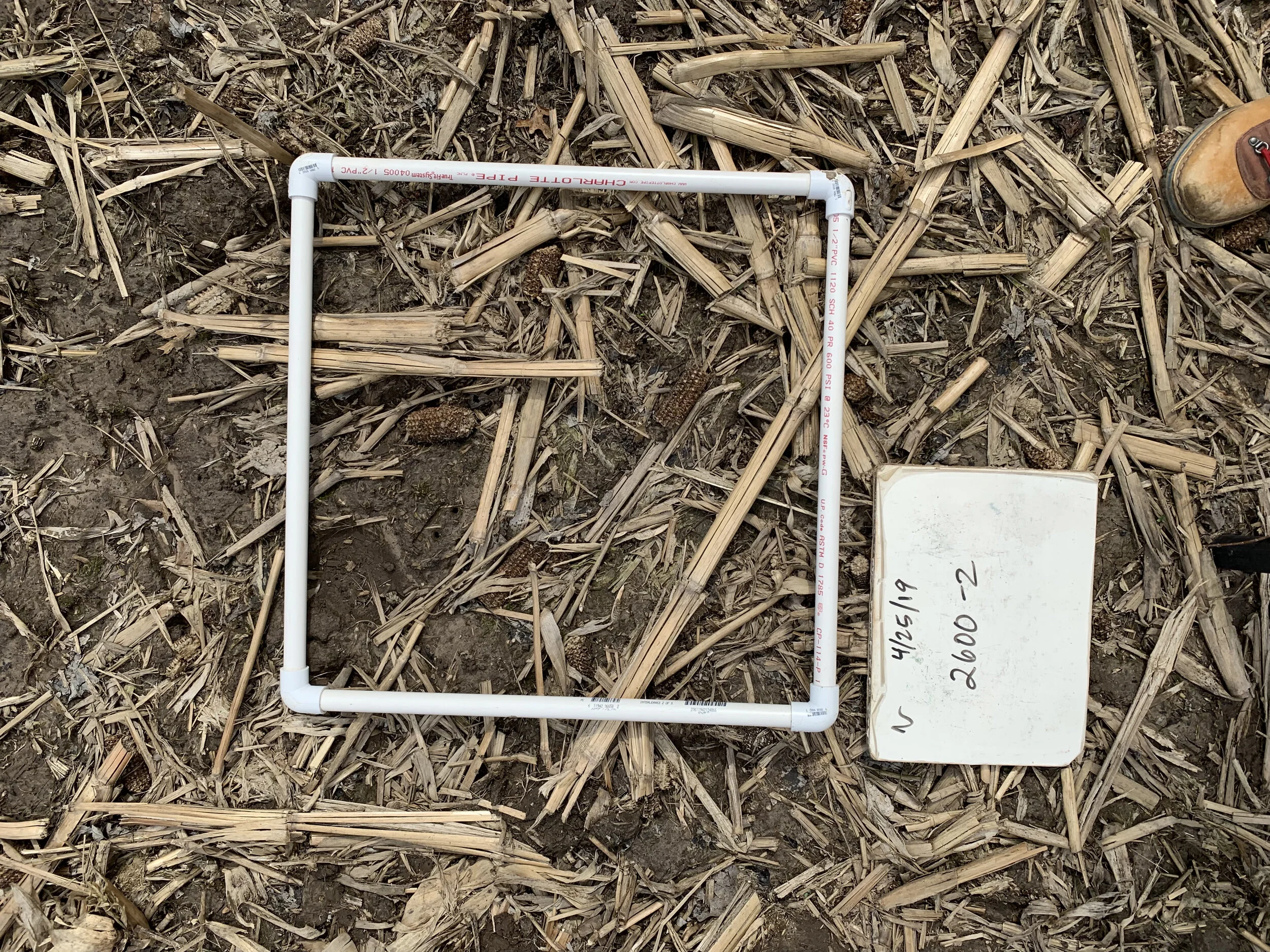

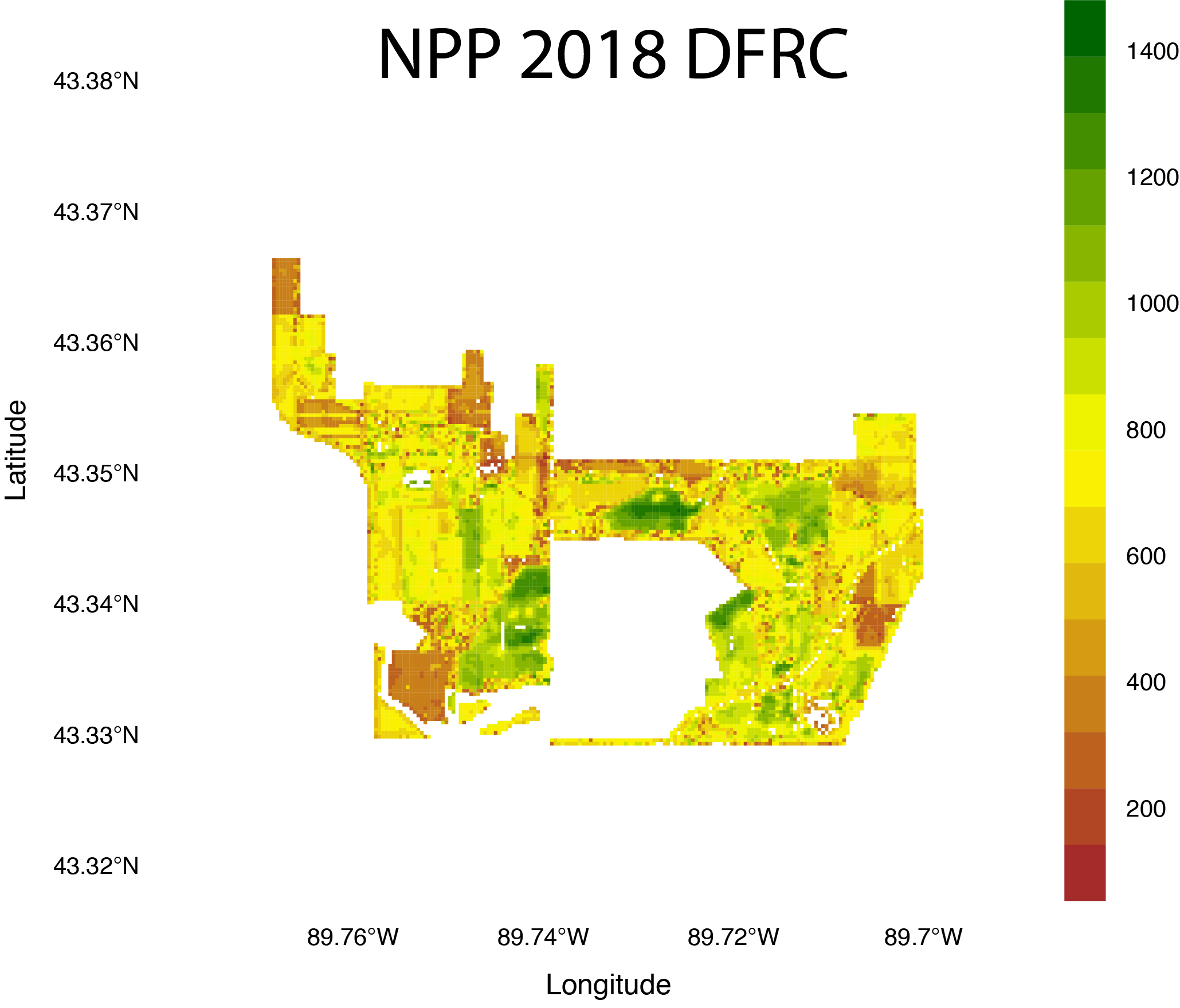